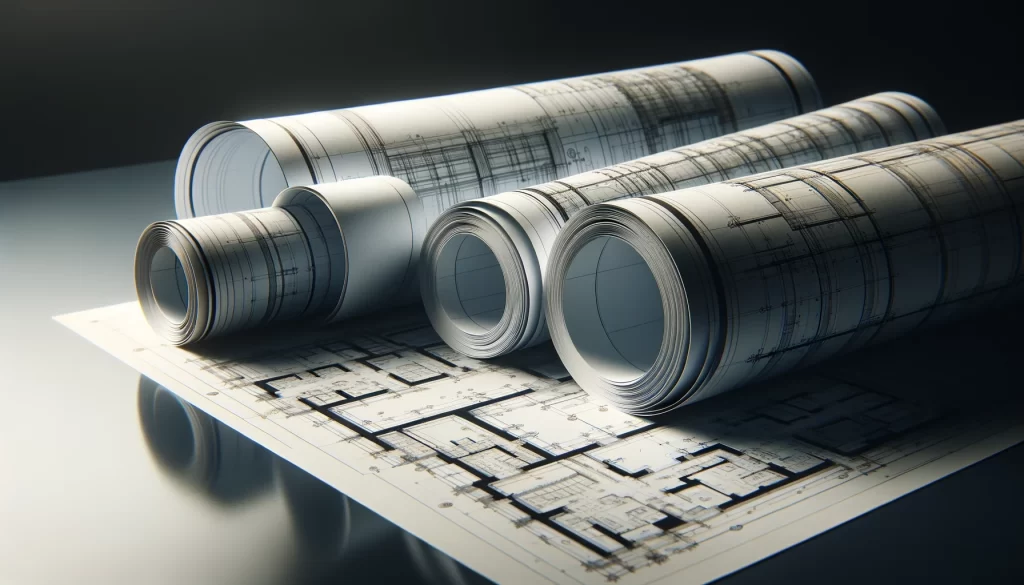
supply chain technology
Blueprints
Supplychain360 blueprints offer an extensive collection of toolkits enabling swift access to best practice to enhance operations or to enable robust decision making.
Addressing supplier risk is increasingly important as global supply chains face unprecedented volatility and disruption. This guide provides a strategic approach to integrating predictive analytics into your risk management practices, significantly enhancing your ability to anticipate and mitigate risks before they impact your operations.
By following this blueprint, you will not only improve supply chain resilience but also achieve greater operational efficiency and cost-effectiveness. Embrace these insights to stay ahead in a competitive and complex market environment.
Enhanced Implementation Steps for Predictive Supplier Risk Management
Step 1: Data Collection and Integration
Identify Relevant Data Sources: Assess data relevance from procurement databases, ERP systems, third-party risk assessment tools, and industry reports. Include innovative sources such as social media for real-time sentiment analysis and news feeds for geopolitical and economic events.
Data Acquisition Techniques: Implement advanced data acquisition tools like real-time data streaming, and IoT device feeds for continuous data collection.
Data Cleansing and Preparation: Utilize automated data cleansing tools to handle large datasets efficiently. Establish a data governance framework to maintain data quality over time.
Data Integration Techniques: Leverage modern data integration platforms (e.g., Apache Nifi, Talend) that support complex transformations and real-time data processing. Apply data federation techniques for access to disparate data without centralizing it physically, ensuring flexibility and scalability.
Data Security and Compliance: Incorporate advanced encryption and anonymization techniques to enhance data security. Develop a compliance checklist specific to different geographical operations to handle data across borders.
Step 2: Model Development
Predictive Modeling Techniques Selection: Evaluate and select from cutting-edge machine learning algorithms based on predictive performance and computational efficiency (e.g., XGBoost, LightGBM).
Building and Configuring Predictive Models: Utilize autoML platforms (e.g., Google AutoML, DataRobot) to automate model selection and tuning processes. Implement model explainability frameworks (e.g., LIME, SHAP) to increase transparency of model predictions, which is crucial for stakeholder trust.
Feature Selection and Engineering: Use advanced feature selection algorithms to identify the most impactful predictors with techniques such as recursive feature elimination (RFE). Integrate time series forecasting methods for dynamic risk factors like market prices or supply chain delays.
Step 3: Model Testing and Validation
Advanced Validation Techniques: Introduce advanced statistical techniques such as Monte Carlo simulations to understand model behavior under various hypothetical scenarios. Use adversarial testing to ensure models are robust against potential data manipulations or unexpected conditions.
Performance Metrics Optimization: Include cost-sensitive learning approaches to weigh predictions based on their potential impact on the supply chain.
Step 4: Deployment and Monitoring
Deployment Strategies: Discuss cloud vs. on-premises deployment advantages and considerations, emphasizing scalability and security aspects. Integrate models into business processes with workflow automation tools like BPM systems to trigger actions based on predictions.
Real-Time Monitoring and Incident Response: Set up an operational incident response team equipped with decision-making protocols when risk thresholds are triggered.
Step 5: Continuous Improvement
Incorporate Feedback Mechanisms: Design a structured feedback mechanism using enterprise collaboration tools to capture qualitative insights from model users.
Sustainability Considerations: Assess environmental impact by incorporating sustainability metrics into supplier evaluations.
Ongoing Learning and Adaptation: Establish a continuous learning framework for the models to adapt to new data without manual reconfiguration, using online learning techniques.
Best Practices for Implementing Predictive Analytics in Supply Chain Operations
Implementing predictive analytics within supply chain operations can significantly enhance decision-making and risk management. Here are some best practices that supply chain leaders should follow to ensure successful implementation and ongoing effectiveness:
Foster a Data-Driven Culture: Encourage all levels of the organization to rely on data for decision-making. This cultural shift requires training and development programs to enhance data literacy across the company.
Ensure Cross-Departmental Collaboration: Effective predictive analytics relies on the integration of data across various departments. Establish collaborative processes that ensure information flows seamlessly between procurement, finance, operations, and sales to create a unified data ecosystem.
Invest in Scalable Technology Infrastructure: As predictive models become more integral to operations, it’s crucial to invest in scalable technology that can handle increased data loads and complex analytics without performance degradation.
Regularly Update Data Sources and Models: The supply chain environment is continuously evolving. Regular updates to data sources and predictive models are necessary to adapt to changes such as new market conditions, supply chain disruptions, or changes in consumer behavior.
Maintain Rigorous Model Testing and Validation: Before deploying predictive models, thoroughly test them using historical and real-time data to ensure they perform accurately under various scenarios. Continuous validation is key to maintaining trust in these tools.
Develop Comprehensive Risk Management Strategies: Use predictive analytics not just for identifying risks but also for developing robust risk management strategies. This involves scenario planning and establishing risk mitigation protocols based on predictive insights.
Prioritize Transparency and Compliance: Given the global nature of supply chains, ensuring compliance with international data protection regulations (like GDPR) is critical. Transparent practices around data usage and model decisions build trust and protect against legal and reputational risk.
Measure Impact and ROI: Establish clear metrics to measure the impact of predictive analytics on supply chain performance. This will help justify continued investment in analytics capabilities and focus efforts on areas with the highest return on investment.
By adhering to these best practices, supply chain leaders can maximize the benefits of predictive analytics, ensuring more proactive and resilient supply chain operations.
Key Metrics and KPIs for Measuring Success in Predictive Supplier Risk Management
To effectively measure the impact and success of predictive analytics in supplier risk management, supply chain directors should focus on a set of precise Key Performance Indicators (KPIs). These metrics provide insights into both the operational efficiency and strategic value of the predictive models implemented:
Predictive Accuracy: Measures how closely the model’s predictions align with actual outcomes. Track this by comparing predicted risks with realized events over a specific period. This metric is crucial for assessing the reliability of the models in real-world scenarios.
Risk Mitigation Rate: Evaluates the effectiveness of actions taken based on predictive insights. This is calculated by the percentage reduction in negative outcomes due to proactive measures taken from model predictions. It reflects the practical value of predictive analytics in preventing disruptions.
Time to Response: Tracks the time it takes for the organization to respond to a risk once it is identified by the predictive model. A shorter response time indicates a more agile and effective supply chain.
Cost Savings: Quantifies the financial impact of using predictive models in terms of reduced losses from avoided risks and improved supply chain efficiency. This can be tracked through comparative cost analyses before and after model implementation.
Supplier Performance Improvement: Measures improvements in supplier reliability and quality as a result of targeted interventions based on model predictions. This KPI can be assessed through regular supplier scorecard reviews.
These KPIs should be regularly monitored through dashboards that integrate real-time data feeds. By interpreting these metrics, supply chain directors can gauge the performance and refine their predictive risk management strategies, ensuring that the supply chain remains robust against potential disruptions.
Overcoming Implementation Challenges in Predictive Analytics for Supplier Risk Management
Integration with Existing Systems:
Challenge: Integrating new predictive analytics tools into existing IT infrastructure without disrupting ongoing operations.
Solution: Adopt a phased implementation approach. Start with a pilot program to assess compatibility and make incremental changes. Utilize middleware to ensure smooth data flow between new analytics systems and existing ERP or SCM systems.
Data Quality and Consistency:
Challenge: Ensuring the quality and consistency of data collected from diverse sources, which is crucial for the accuracy of predictive models.
Solution: Implement robust data governance policies. Use advanced data cleansing and validation tools to automate the process of identifying and correcting data discrepancies. Regularly audit data processes to maintain high data quality standards.
Change Management:
Challenge: Resistance from within the organization due to changes in workflows and processes.
Solution: Conduct comprehensive training sessions and workshops to demonstrate the benefits of predictive analytics. Develop change champions within each department who can advocate for and guide the transition.
Scalability and Flexibility:
Challenge: Ensuring that predictive systems can scale with the growth of the business and adapt to new challenges as they arise.
Solution: Opt for scalable cloud solutions that allow for the addition of resources as needed. Design models with modularity to easily adjust or replace components without overhauling the entire system.
Legal and Regulatory Compliance:
Challenge: Adhering to international data protection and privacy laws, especially when operating across multiple countries.
Solution: Involve legal experts early in the design process to ensure all data handling procedures comply with relevant laws like GDPR. Use data anonymization and encryption to protect sensitive information.
Maintaining Model Relevance:
Challenge: Keeping predictive models relevant in the face of rapidly changing market conditions and external variables.
Solution: Establish a continuous learning strategy for models to incorporate new data and insights regularly. Utilize adaptive learning systems that automatically adjust to changes in data patterns.
By addressing these challenges with effective strategies, supply chain leaders can enhance their capabilities in predictive analytics, leading to improved risk management and operational efficiencies. This proactive approach ensures that the supply chain is resilient, adaptive, and aligned with business objectives.
This blueprint equips you with advanced tools to proactively manage supplier risks, boosting your supply chain’s resilience and efficiency. By adopting these strategies, you ensure your operations remain robust, agile, and competitive in a dynamically evolving global marketplace.
Unlock full access to a wealth of expert insights by subscribing to SupplyChain360. Dive into our extensive library of blueprints and resources designed to elevate your supply chain strategies. Don’t miss out—subscribe today and lead your supply chain to new heights of efficiency and innovation.