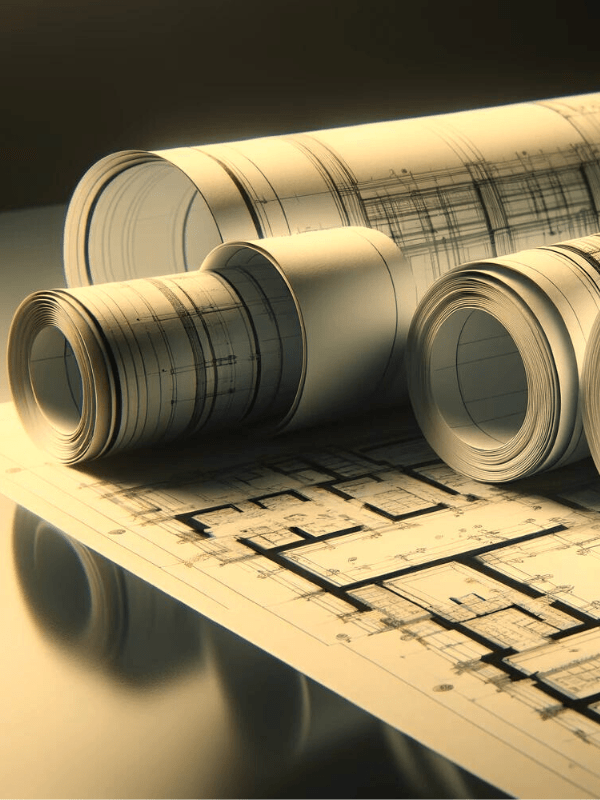
supply chain operations series
Transport & Logistics
BLUEPRINTS
This blueprint presents a critical pathway to transforming fleet operations through predictive maintenance strategies, tackling the challenge head-on by leveraging cutting-edge technologies and data analytics.
By adopting the methodologies outlined here, supply chain directors can anticipate issues before they escalate, ensuring operational continuity and significant cost savings. This blueprint not only guides you through the implementation of predictive maintenance but also equips you with the tools to optimize fleet performance and stay ahead in the competitive landscape of global logistics.
Implementing a predictive maintenance strategy for transportation fleets involves several key steps, each requiring careful planning and execution. By following this guide, fleet managers can transition from traditional reactive maintenance approaches to a more efficient and cost-effective predictive maintenance regime.
Step 1: Data Collection
Vehicle Sensor Installation:
- Equip vehicles with IoT sensors to monitor critical components (e.g., engine performance, tire pressure, brake system).
- Ensure sensors cover a wide range of diagnostics to provide comprehensive data.
Telematics Integration:
- Implement telematics systems to collect real-time data on vehicle location, fuel consumption, and driver behavior.
- Choose telematics solutions that offer seamless integration with existing fleet management software.
Data Aggregation Framework:
- Establish a centralized data repository to aggregate data from various sources.
- Utilize cloud storage solutions for scalability and remote access.
Step 2: Analysis and Integration
Predictive Analytics Platform Selection:
- Deploy a predictive analytics platform capable of processing large volumes of data.
- Consider platforms that support machine learning algorithms and offer user-friendly interfaces for non-technical staff.
Machine Learning Model Development:
- Develop or customize machine learning models tailored to the specific needs and patterns of your fleet.
- Use historical maintenance records and real-time data to train models, focusing on identifying patterns that precede failures.
Integration with Maintenance Planning:
- Ensure the analytics platform can integrate with maintenance scheduling tools to automate the planning process.
- Establish thresholds for alerts that trigger maintenance actions to prevent downtime.
Step 3: Strategy Development
Maintenance Scheduling Based on Predictions:
- Develop a dynamic maintenance schedule that adjusts based on predictive analytics insights.
- Prioritize maintenance tasks based on the criticality of potential failures and their impact on operations.
Resource Allocation Optimization:
- Use predictions to optimize the allocation of maintenance resources, including personnel and spare parts.
- Implement just-in-time inventory practices for spare parts based on predictive needs assessments.
Risk Management Planning:
- Incorporate risk assessment into the maintenance strategy to address the likelihood and impact of potential failures.
- Develop contingency plans for scenarios where critical failures might occur despite predictive maintenance efforts.
Step 4: Execution
Staff Training and Development:
- Conduct training sessions for maintenance staff on new technologies and predictive maintenance procedures.
- Foster a culture of continuous learning and improvement to keep pace with technological advancements.
Predictive Maintenance Task Implementation:
- Execute maintenance tasks as planned, focusing on preemptive repairs and adjustments to prevent predicted failures.
- Ensure maintenance activities are documented and analyzed for continuous improvement.
Technology and Process Evaluation:
- Regularly review the performance of predictive maintenance technologies and processes.
- Adjust models and strategies based on feedback and new data to enhance accuracy and efficiency.
Step 5: Feedback Loop
Performance Monitoring and Analysis:
- Monitor the outcomes of predictive maintenance actions, including changes in vehicle uptime, maintenance costs, and incident rates.
- Use data visualization tools to present key performance indicators (KPIs) and insights.
Continuous Improvement Process:
- Implement a structured process for continuous improvement, utilizing methodologies like PDCA (Plan-Do-Check-Act) or Six Sigma.
- Encourage feedback from all stakeholders, including maintenance staff, drivers, and management, to inform process enhancements.
Innovation and Adaptation:
- Stay informed about emerging technologies and best practices in predictive maintenance and fleet management.
- Be prepared to adapt and innovate the maintenance strategy in response to new opportunities and challenges.
By meticulously following these steps and focusing on the integration of advanced technologies with data-driven decision-making processes, fleet managers can significantly enhance the reliability and efficiency of their transportation fleets. This predictive maintenance guide serves as a blueprint for minimizing downtime, reducing maintenance costs, and optimizing fleet operations in a competitive landscape.
For supply chain directors, it’s essential to measure the impact of predictive maintenance on fleet operations. The following key metrics and KPIs offer a clear picture of the strategy’s effectiveness:
- Downtime Reduction: This metric tracks the decrease in vehicle unavailability due to maintenance issues. It’s calculated by comparing the duration of unplanned downtime before and after implementing predictive maintenance. A significant reduction indicates the strategy’s success in preventing unexpected failures.
- Maintenance Cost Savings: This KPI measures the change in maintenance expenses, highlighting the cost-effectiveness of predictive maintenance. Savings can result from avoiding major repairs, extending vehicle lifespans, and optimizing spare parts inventory. It’s essential to track both direct and indirect costs, including those related to downtime and lost productivity.
- Return on Investment (ROI): ROI quantifies the financial return from predictive maintenance initiatives. It considers the total cost of implementation, including technology investments and training, against the savings achieved through reduced downtime and maintenance costs. A positive ROI signifies that the benefits of predictive maintenance outweigh the costs.
- Equipment Lifespan Extension: This metric assesses the increase in the operational lifespan of fleet vehicles and equipment due to improved maintenance practices. Longer lifespans mean lower replacement costs and higher asset value, contributing to overall fleet efficiency.
Tracking these metrics requires integrating data from various sources, including maintenance records, operational logs, and financial reports. Advanced analytics tools can help interpret this data, providing insights into trends and areas for improvement. Regularly reviewing these KPIs enables supply chain directors to fine-tune their predictive maintenance strategies, ensuring continuous optimization of fleet operations.
To successfully implement a predictive maintenance strategy in supply chain operations, leveraging the right tools and technologies is crucial. These tools not only facilitate the collection and analysis of data but also enable proactive decision-making to prevent equipment failure and optimize fleet performance.
- IoT Sensors: Internet of Things (IoT) sensors play a foundational role in predictive maintenance by collecting real-time data from fleet vehicles. These sensors can monitor a wide range of parameters, including temperature, vibration, and wear, providing the raw data necessary for predictive analysis.
- Telematics Systems: Telematics technology offers comprehensive monitoring of vehicle performance, including location tracking, fuel consumption, and driver behavior. This data is integral to understanding the operational conditions of the fleet and identifying potential issues before they lead to breakdowns.
- Predictive Analytics Platforms: These platforms analyze the vast amounts of data gathered by IoT sensors and telematics systems. They utilize machine learning algorithms to identify patterns and predict potential failures. Features such as customizable dashboards and alert systems help in planning maintenance schedules and allocating resources efficiently.
- Cloud Computing: Cloud services offer scalable data storage and processing capabilities, essential for managing the large volumes of data generated by a fleet. Cloud platforms also facilitate integration with other supply chain management systems, ensuring a seamless flow of information across the organization.
- Maintenance Management Software: This software streamlines the scheduling, tracking, and execution of maintenance tasks. It can integrate with predictive analytics platforms to automate maintenance planning based on predictive insights, ensuring timely intervention before equipment failures occur.
- Data Visualization Tools: These tools present complex data in an accessible format, making it easier for decision-makers to understand trends, performance metrics, and predictive insights. Effective data visualization supports informed decision-making and continuous improvement in maintenance strategies.
Integrating these tools and technologies into the predictive maintenance process enhances the ability to anticipate and prevent equipment failures, reduce downtime, and optimize maintenance resources. They form the technological backbone of a predictive maintenance strategy, enabling supply chain directors to maintain operational efficiency and competitiveness in a fast-paced market.
Implementing predictive maintenance within supply chain operations requires a strategic approach to ensure maximum efficiency and effectiveness. Here are some best practices to guide this implementation:
- Embrace a Data-Driven Culture: Encourage decision-making based on data analysis and insights across all levels of the organization. This involves not only collecting data but also ensuring it is accessible and actionable for relevant stakeholders.
- Invest in Training and Development: Equip your team with the necessary skills to handle new technologies and methodologies associated with predictive maintenance. Continuous education on the latest trends and tools in predictive maintenance is crucial for keeping your operations at the forefront of efficiency.
- Integrate Systems Seamlessly: Ensure that your predictive maintenance tools and platforms are fully integrated with other supply chain management systems. This integration allows for smoother operations and enhances the ability to make informed decisions based on a comprehensive view of supply chain activities.
- Prioritize Communication and Collaboration: Foster strong communication channels between different departments (e.g., IT, operations, maintenance) to ensure that predictive maintenance insights are shared and acted upon effectively. Collaboration also helps in fine-tuning the predictive models based on cross-functional insights.
- Set Clear Metrics and Goals: Define specific, measurable goals for your predictive maintenance program, such as reducing unplanned downtime, extending asset life, or improving maintenance response times. Use these metrics to track progress and make adjustments as needed.
- Implement Incrementally: Start with a pilot program focusing on a critical segment of your operations. This allows you to measure the impact, gather insights, and make necessary adjustments before rolling out the program across the entire supply chain.
- Stay Agile and Adapt: The supply chain environment is dynamic, with evolving challenges and opportunities. Stay open to adapting your predictive maintenance strategies in response to new technologies, market conditions, and operational insights.
By adhering to these best practices, supply chain directors can ensure a smoother transition to predictive maintenance, leading to enhanced operational efficiency, reduced costs, and improved service levels within their supply chain operations.
-
High Initial Investment Costs: The upfront cost of predictive maintenance technologies, including IoT sensors, analytics software, and training, can be significant.Solution: Conduct a thorough cost-benefit analysis to understand the long-term savings and ROI. Explore phased implementation and leasing options for expensive equipment to spread out costs.
-
Complexity of Data Integration: Integrating data from diverse sources and systems can be complex and time-consuming.Solution: Invest in interoperable systems and platforms with robust API capabilities. Consider hiring or partnering with data integration specialists to streamline the process.
-
Skill Gaps and Training Needs: The transition to predictive maintenance often requires new skills and competencies that the existing workforce may not possess.Solution: Develop a comprehensive training program focusing on new technologies and predictive maintenance techniques. Foster partnerships with technology providers for specialized training and support.
-
Resistance to Change: Changing from a traditional to a predictive maintenance approach can meet resistance from staff accustomed to the existing processes.Solution: Implement change management strategies, including clear communication of benefits, involving staff in the transition process, and addressing concerns proactively.
-
Reliability of Predictive Models: Predictive models are only as good as the data and algorithms they are based on, and inaccuracies can lead to mistrust.Solution: Regularly review and refine predictive models with the latest data and feedback. Start with pilot projects to build confidence in the models before full-scale implementation.
-
Cybersecurity Risks: The increased reliance on digital technologies and data connectivity raises the risk of cyber threats.Solution: Prioritize cybersecurity from the outset, implementing strong data protection measures and regularly updating security protocols.
-
Scalability Issues: As the supply chain grows, the predictive maintenance system must be able to scale accordingly without loss of performance.Solution: Choose scalable technologies and platforms from the beginning. Regularly assess system performance and scalability needs as part of the continuous improvement process.
By embracing this blueprint for predictive maintenance, you’ll unlock unparalleled operational efficiency and cost savings within your fleet. It’s your roadmap to staying ahead, ensuring your fleet operations are not just reactive, but proactively optimized for the challenges of tomorrow’s supply chain landscape.
Unlock the full potential of your supply chain with SupplyChain360. Subscribe now for exclusive access to more blueprints, cutting-edge strategies, and the resources you need to lead in logistics.