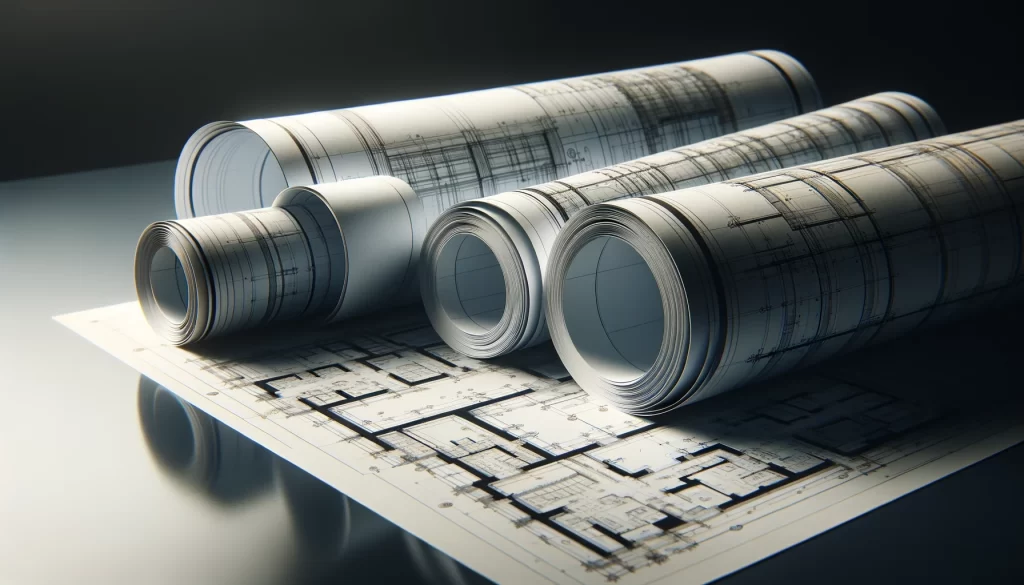
supply chain strategy
Blueprints
Supplychain360 blueprints offer an extensive collection of toolkits enabling swift access to best practice to enhance operations or to enable robust decision making.
This blueprint on integrating predictive analytics for strategic supplier selection addresses the critical challenge of optimizing supplier networks in a dynamic global market. The strategic application of predictive analytics empowers you to enhance decision-making, reduce risks, and increase operational efficiencies.
By implementing the steps and strategies detailed here, you’ll gain the ability to anticipate market changes, align supply chain practices with business objectives, and achieve a competitive advantage. Utilizing this blueprint ensures not only cost reduction but also improvements in supplier performance and resource management—key to thriving in today’s complex supply environments.
Implementation Steps
This comprehensive guide is structured to provide clear, actionable steps for each phase of implementing predictive analytics in supplier selection, ensuring that supply chain leaders can achieve enhanced decision-making capabilities with high standards of accuracy and efficiency.
Step 1: Define Business Objectives and Supplier Requirements
Identify Key Objectives: Determine precise objectives for predictive analytics in supplier selection such as reducing costs, enhancing supplier reliability, improving product quality, and achieving sustainability goals.
Determine Supplier Criteria: Establish detailed supplier selection criteria, including but not limited to financial stability, delivery performance, quality management systems, innovation capabilities, and ethical standards.
Align Objectives with Business Strategy: Ensure that the selection criteria align with the company’s strategic goals like market expansion, innovation, sustainability commitments, and cost leadership.
Develop Weighting Systems: Create a scoring system for supplier criteria to quantify priorities and facilitate data-driven decision-making.
Step 2: Data Collection and Management
Data Sources Identification: Identify and catalog available internal and external data sources. Internally, this may include procurement databases, quality management logs, and contract compliance reports. Externally, look to industry reports, financial statements, and sustainability indexes.
Data Collection Tools: Implement tools such as ERP and CRM systems, supplemented with web scraping for real-time market data and APIs for continuous data streams.
Data Quality Assurance: Regularly perform data cleansing, normalization, and transformation processes to ensure high data quality and usability.
Establish Data Governance: Create a framework for data governance that addresses data accuracy, access controls, regulatory compliance, and ethical considerations.
Step 3: Developing Predictive Models
Select Modeling Techniques: Choose from a range of predictive modeling techniques based on the nature of the data and the complexity of the selection criteria. Techniques might include logistic regression, random forests, support vector machines, or neural networks.
Model Development: Utilize platforms like TensorFlow or PyTorch for building complex models, or R and Python for statistical models. Incorporate cross-validation techniques to avoid overfitting.
Feature Selection: Employ techniques like principal component analysis (PCA) or feature importance scoring to determine the most relevant variables for supplier performance prediction.
Model Training and Testing: Split data into training, validation, and test sets. Use the training set to build the model and the validation set to tune parameters. Evaluate model performance on the test set to ensure it generalizes well to new data.
Step 4: Testing and Validation
Backtesting: Test the model using historical data from past supplier engagements to compare the model’s predictions with actual outcomes.
Validation Metrics: Apply statistical metrics such as ROC-AUC, confusion matrix, mean absolute error, and others to evaluate the model’s predictive accuracy and reliability.
Stakeholder Review: Organize workshops with key stakeholders to review predictive outcomes, gather insights, and ensure that model results align with business expectations.
Iterative Refinement: Refine the model iteratively based on stakeholder feedback and ongoing monitoring of model performance in live environments.
Step 5: Integration into Procurement Processes
Workflow Integration: Seamlessly integrate predictive analytics outputs into procurement software systems through custom APIs or middleware solutions.
Decision Support Systems: Develop interactive dashboards using BI tools like Tableau or Power BI that provide visualizations of supplier assessments and predictive scores.
Training and Support: Develop comprehensive training programs for procurement teams that cover analytical techniques, interpretation of model outputs, and decision-making protocols based on predictive analytics.
Step 6: Continuous Improvement and Feedback Loop
Monitor Outcomes: Establish regular review cycles to assess the impact of predictive analytics on supplier selection and overall supply chain performance.
Feedback Mechanisms: Implement structured feedback mechanisms to capture user experiences and suggestions for improvement of the predictive system.
Ongoing Model Tuning: Regularly update and refine models with new data and insights, adapting to changes in market conditions and internal business strategies.
Reporting and Documentation: Maintain detailed documentation of model updates, performance reports, and business outcomes to ensure transparency and support continuous learning and improvement.
This structured, detailed guide provides a robust framework for integrating predictive analytics into supplier selection processes. Each step is clearly defined with actionable points and modern analytical tools and frameworks, ensuring that supply chain leaders can effectively apply predictive analytics to enhance decision-making and supplier management.
Best Practices
To ensure successful implementation of predictive analytics in supply chain operations, supply chain leaders should adhere to several best practices. These practices not only facilitate the integration of advanced analytics but also maximize the potential benefits.
Establish a Cross-Functional Team
Team Composition: Form a cross-functional team comprising members from procurement, IT, operations, and finance. This diversity ensures a holistic approach to implementing predictive analytics.
Roles and Responsibilities: Clearly define each team member’s role, focusing on leveraging their expertise to support different aspects of the analytics implementation.
Develop a Strong Data Culture
Promote Data Literacy: Invest in training programs to enhance data literacy across the organization. Everyone from executives to front-line employees should understand the basics of data handling and interpretation.
Data-Driven Decision-Making: Encourage decisions based on data and analytics rather than intuition. This shift may require cultural changes within the organization.
Prioritize Data Quality
Data Accuracy: Regular audits and validations of data sources and datasets are crucial. Accurate data is the backbone of reliable predictive analytics.
Data Integration: Ensure seamless integration of data from various sources. This might involve upgrading IT infrastructure or adopting new technologies that facilitate better data aggregation and accessibility.
Implement Scalable Analytical Solutions
Scalability of Tools: Choose analytical tools and solutions that can scale with your business. As your supply chain operations grow, your analytics tools should adapt and manage increased data volumes and complexity without performance loss.
Flexibility in Processes: Be prepared to modify workflows and processes as new trends and technologies emerge. Adaptability ensures that your supply chain remains resilient and competitive.
Continuous Improvement and Innovation
Feedback Loops: Establish mechanisms for continuous feedback on analytics processes and outcomes. This feedback is vital for ongoing improvement.
Innovate Actively: Stay abreast of advancements in analytics and machine learning. Experiment with new tools and techniques to continuously refine your predictive capabilities.
By following these best practices, supply chain leaders can create a robust environment where predictive analytics not only thrives but drives significant improvements in supplier selection and overall supply chain performance. This approach ensures a strategic advantage in today’s competitive market, enabling more informed decision-making and enhanced operational efficiency.
Key Metrics and KPIs
To effectively measure the impact of predictive analytics on supply chain operations, supply chain leaders should focus on a set of key performance indicators (KPIs). These metrics provide actionable insights and help gauge the effectiveness of implemented strategies.
Supplier Performance Index (SPI)
Supplier Performance Index (SPI): This index evaluates suppliers based on various criteria such as delivery time, quality, cost, and compliance. Track SPI by collecting data from ERP systems and scorecards, analyzing trends and identifying areas for improvement.
Inventory Turnover Ratio
Inventory Turnover Ratio: A critical measure of how efficiently inventory is managed and sold over time. It can be calculated by dividing the cost of goods sold by the average inventory. An increase in this ratio typically indicates improved efficiency in inventory management, suggesting successful predictive modeling in procurement and stock management.
Order Accuracy Rate
Order Accuracy Rate: Measures the accuracy of order fulfillment. This is tracked by comparing the number of orders shipped without errors to the total orders shipped. High accuracy rates often reflect the effective use of predictive analytics in forecasting and planning.
Lead Time Reduction
Lead Time Reduction: Evaluates the effectiveness of predictive analytics in reducing the time from order to delivery by analyzing the time series data before and after implementation. Reduction in lead time can significantly enhance customer satisfaction and operational efficiency.
Cost Reduction Percentage
Cost Reduction Percentage: This KPI tracks the percentage reduction in operational costs resulting from improved supplier selection and inventory management. It highlights the financial impact of predictive analytics and can be measured by comparing cost data pre- and post-implementation.
Sustainability Index
Sustainability Index: For companies focusing on environmental impact, this index measures improvements in sustainability practices, like reduced carbon footprint and waste, through better supplier selection and optimized logistics.
By regularly monitoring these KPIs, supply chain directors can not only assess the effectiveness of predictive analytics but also refine their strategies for continuous improvement. The interpretation of these metrics should involve cross-departmental reviews to ensure a comprehensive understanding of their implications on the overall business objectives.
Implementation Challenges
Implementing predictive analytics in supply chain management can present several challenges, but with the right strategies, these can be effectively mitigated. Here’s a detailed look at common obstacles and practical solutions to ensure successful implementation:
Data Quality and Integration
Challenge: Poor data quality and difficulty in integrating data from various sources can hinder the effectiveness of predictive analytics.
Solution: Implement rigorous data governance policies to ensure data accuracy and consistency. Invest in integration tools that can consolidate data across different systems and formats, ensuring a unified view that facilitates deeper analysis.
Resistance to Change
Challenge: Resistance from employees, particularly from those accustomed to traditional methods, can slow down or derail new technology implementations.
Solution: Conduct comprehensive training sessions to demonstrate the benefits of predictive analytics. Engage employees early in the process through workshops and seminars that allow them to see the practical benefits of their contributions to data-driven decision-making.
Technology Adaptation and Scalability
Challenge: Finding the right technology that fits the company’s current systems and is scalable for future needs can be daunting.
Solution: Start with a pilot project to test different technologies before full-scale implementation. Choose platforms that are known for their scalability and compatibility with existing systems.
Cost Management
Challenge: The initial investment in technology and training can be substantial, making it difficult to obtain buy-in from upper management.
Solution: Present a detailed ROI analysis that outlines the long-term savings and efficiency gains from predictive analytics. Highlight case studies and benchmarks from other companies that have successfully implemented similar technologies.
Maintaining Data Security
Challenge: With the increased use of data analytics, securing sensitive information becomes a paramount concern.
Solution: Implement state-of-the-art cybersecurity measures and regular audits to protect data integrity and privacy. Train staff on the importance of data security and the role they play in maintaining it.
Continuous Improvement
Challenge: Ensuring continuous improvement and keeping the system updated with the latest data and analytics techniques can be challenging.
Solution: Establish a dedicated team responsible for the ongoing evaluation of the analytics system. This team should stay informed about advances in analytics technologies and methodologies, and integrate them into the existing system regularly.
By anticipating these challenges and preparing solutions in advance, supply chain leaders can enhance the success rate of predictive analytics implementation, leading to better decision-making, increased efficiency, and competitive advantage in the marketplace.
This blueprint equips you to leverage predictive analytics for strategic supplier selection, enhancing efficiency and reducing costs. By adopting its strategies, you position your supply chain for resilience and competitive advantage, ready to meet the demands of a rapidly evolving marketplace.
Unlock the full potential of your supply chain with exclusive access to our expertly crafted blueprints and resources. Subscribe to SupplyChain360 today and stay ahead of industry trends, ensuring your operations are optimized for success. Don’t miss out—join our community of leaders now!