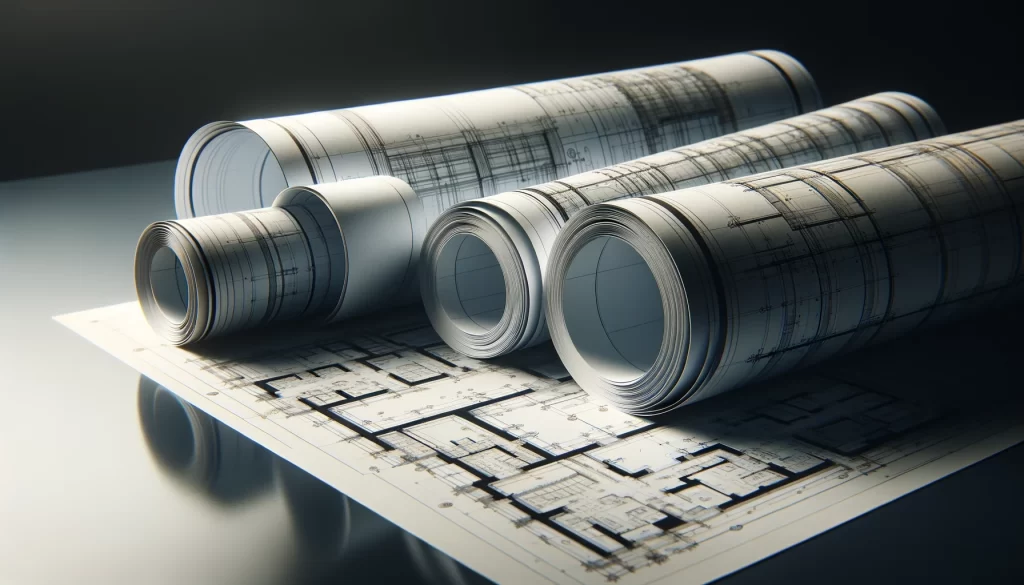
TECHNOLOGY
Blueprints
This blueprint provides a comprehensive guide for integrating advanced analytics into supplier selection, offering actionable steps, best practices, and key metrics to enhance supply chain operations.
Supply chain leaders face the pressing challenge of optimizing supplier selection through sophisticated data analysis. This blueprint on integrating advanced analytics for supplier selection provides strategic methodologies crucial for enhancing decision-making capabilities within global supply chains. Focused on actionable insights, it equips supply chain directors with robust analytical tools and step-by-step guidance to elevate procurement strategies and operational efficiency.
Employing this blueprint will not only streamline supplier selection processes but also empower supply chains to adapt swiftly to market changes and emerging risks, ensuring a competitive advantage in a rapidly evolving industry landscape.
Enhanced Implementation Steps: Integrating Advanced Analytics for Strategic Supplier Selection
This section provides an in-depth, comprehensive guide structured to facilitate the successful integration of advanced analytics into supplier selection processes by supply chain leaders. Each main step is elaborated with several sub-steps, incorporating advanced models, frameworks, and industry best practices to ensure the utility and effectiveness of the implementation.
Step 1: Define Objectives and Scope
Objective Setting: Engage key stakeholders to define specific business objectives such as reducing supplier risk, enhancing quality, or optimizing cost structures. Utilize SMART criteria to ensure objectives are Specific, Measurable, Achievable, Relevant, and Time-bound.
Scope Definition: Determine the range of application—whether localized to certain product lines or applied globally across all supplier interactions. Clarify the boundaries of the project regarding analytics reach (e.g., regional vs. global suppliers).
Criteria Development: Develop a set of performance metrics and selection criteria aligned with business objectives. Employ decision matrix tools like Analytical Hierarchy Process (AHP) to weight criteria according to strategic importance.
Step 2: Data Collection and Management
Data Mapping: Conduct a comprehensive mapping of available internal and external data sources. Identify gaps in existing data and plan for acquisition or partnerships necessary to fill these gaps.
Data Integration and Technology: Select and implement robust data integration technologies (e.g., ETL tools). Ensure integration compatibility with existing ERP and SCM systems.
Data Quality Assurance: Establish a continuous data quality management protocol. Use data cleansing and normalization techniques to maintain data integrity.
Step 3: Analytics Model Selection and Development
Model Exploration: Review and select from predictive, prescriptive, and descriptive analytics models based on the defined objectives. Consider machine learning algorithms like random forests or neural networks for dynamic supplier evaluation.
Tool and Platform Selection: Choose advanced analytics platforms that offer scalability, security, and comprehensive analytical capabilities (e.g., SAS, R, Python). Evaluate cloud-based analytics solutions for enhanced collaboration and data sharing.
Framework Implementation: Apply the SCOR model to align supplier performance metrics with overall supply chain objectives. Integrate risk assessment frameworks such as FAIR to quantify and mitigate supplier risks.
Step 4: Pilot Testing and Validation
Prototype Development: Develop a prototype based on selected models to test theories and hypotheses in a controlled environment.
Pilot Implementation: Roll out the analytics solution to a selected group of suppliers or a specific supply chain segment. Monitor the implementation closely to evaluate the effectiveness and accuracy of the analytics insights.
Stakeholder Feedback: Collect and analyze stakeholder feedback to identify any practical or technical issues. Adjust the prototype based on feedback to improve functionality and user experience.
Step 5: Full-scale Implementation
Integration and Deployment: Integrate the analytics solution into the existing procurement and supply chain systems. Develop a detailed deployment roadmap, specifying timelines and milestones.
Training and Support: Design and deliver comprehensive training programs to all relevant users to ensure smooth adoption. Establish a support structure to assist users post-deployment.
Change Management: Implement change management strategies to address resistance and enhance organizational buy-in. Use communication plans to keep all stakeholders informed and engaged.
Step 6: Continuous Monitoring and Optimization
Performance Monitoring: Use dashboards and real-time data visualizations to monitor supplier performance against KPIs. Regularly review analytics outputs to ensure they remain aligned with strategic objectives.
Continuous Improvement: Implement a structured approach for ongoing model refinement and algorithm tuning. Foster a culture of continuous improvement by regularly soliciting and integrating user feedback.
Innovation Adoption: Stay abreast of advancements in analytics and supply chain management technologies. Periodically assess the potential for incorporating new tools or techniques into the existing framework.
Step 7: Feedback Loop and Strategic Review
Feedback Mechanisms: Develop and implement feedback systems to capture user satisfaction and system effectiveness. Use feedback to refine processes and address any emerging challenges.
Strategic Review: Conduct periodic strategic reviews to ensure the analytics initiative remains aligned with evolving business goals. Adapt the strategy based on changes in the business environment, technology advancements, or supplier dynamics.
Best Practices for Advanced Analytics in Supply Chain Operations
Implementing advanced analytics within supply chain operations can transform decision-making processes and enhance operational efficiency. Here are key best practices to follow to ensure successful integration and maximization of benefits from advanced analytics:
1. Establish Clear Data Governance
Develop a robust data governance framework to ensure data accuracy, accessibility, and security. Define clear roles and responsibilities for data management and establish data standards to maintain consistency across all sources.
2. Emphasize Collaborative Planning
Foster collaboration between departments such as procurement, logistics, and IT to integrate analytics seamlessly. Collaboration ensures that analytical insights align with the operational realities and strategic goals of different segments of the supply chain.
3. Adopt Scalable Analytical Solutions
Choose analytics platforms that can scale with your business needs. Consider cloud-based solutions for flexibility and scalability, allowing your supply chain operations to adapt to changes in data volume and computational demands.
4. Invest in Talent and Training
Building a team with skills in data science and analytics is crucial. Invest in training existing staff and recruiting new talent to manage and interpret analytical models effectively. Continuous learning opportunities should be provided to keep the team updated on the latest analytical techniques and tools.
5. Implement Incremental Changes
Start with pilot projects to test the effectiveness of analytics in smaller, controlled environments. Gradual implementation helps in identifying potential issues early and allows for adjustments before a full-scale rollout.
6. Utilize Real-Time Data
Leverage real-time data to enhance responsiveness and agility in the supply chain. Real-time analytics can help in making quick decisions that are critical in dynamic market conditions.
7. Monitor and Continuously Improve
Establish metrics to evaluate the effectiveness of analytics initiatives. Use these insights to continuously refine and optimize the analytics processes. Encourage feedback from all stakeholders to improve the usability and functionality of analytical tools.
By adhering to these best practices, supply chain leaders can better navigate the complexities of integrating advanced analytics into their operations, ensuring that their supply chains are more responsive, efficient, and competitive in the market. These strategies not only align with operational goals but also enhance decision-making capabilities, crucial for maintaining a resilient and agile supply chain.
Key Metrics and KPIs for Measuring Success in Advanced Analytics Integration
To effectively measure the success of advanced analytics integration within supply chain operations, supply chain directors should focus on several critical Key Performance Indicators (KPIs). These metrics not only assess the immediate impact of analytics but also help in steering long-term strategic decisions.
1. Supplier Performance Index (SPI):
This composite index can include metrics such as on-time delivery rate, quality acceptance rate, and response time. Track this using real-time dashboards that aggregate data across various dimensions of supplier performance, enabling quick assessment and response.
2. Cost Savings:
Measure reductions in procurement and logistics costs as a result of analytics-driven supplier selection and management. This can be tracked through year-over-year comparisons of cost metrics relative to the procurement volume.
3. Risk Mitigation:
Evaluate the effectiveness of analytics in identifying and mitigating risks by tracking incidents of supply chain disruptions and their financial impact. Use trend analysis to monitor changes over time and assess the predictive capabilities of your analytics tools.
4. Supply Chain Agility:
Measure the speed of response to market changes and supply chain disruptions. Key metrics include the time to source alternate suppliers and the lead time reductions achieved through analytics-enhanced processes.
5. Innovation and Improvement Rate:
Track the rate of improvement or innovation in supply chain processes facilitated by analytics. This includes new strategies for logistics, improved supplier collaboration models, or technology upgrades.
Each of these KPIs should be regularly reviewed using statistical tools and business intelligence software to ensure accuracy and relevance. Supply chain directors should also consider setting benchmarks based on industry standards or historical company performance to contextualize the data. Regularly revisiting these benchmarks and adjusting them based on new insights and changing business conditions is crucial for maintaining a competitive edge and achieving continuous improvement in supply chain operations.
Overcoming Implementation Challenges in Advanced Analytics for Supply Chains
Integrating advanced analytics into supply chain operations can encounter several challenges. Understanding these obstacles and preparing solutions in advance can significantly enhance the success rate of analytics projects. Below are common challenges faced when implementing advanced analytics in supply chains, along with practical solutions for each.
1. Data Quality and Integration Issues:
Challenge: Inconsistent data quality and integration difficulties from disparate systems can hinder analytics effectiveness.
Solution: Establish a robust data governance framework to standardize data collection and ensure consistent quality. Use advanced data integration tools that support diverse formats and sources, facilitating smoother data consolidation.
2. Resistance to Change:
Challenge: Organizational resistance from employees accustomed to traditional processes can obstruct the adoption of new technologies.
Solution: Foster a culture of innovation through continuous education and training programs. Clearly communicate the benefits of analytics to all stakeholders to increase buy-in and reduce resistance.
3. Skill Gaps:
Challenge: Lack of in-house expertise in data science and analytics can pose a significant barrier.
Solution: Invest in training current employees and consider hiring new talent with specific analytics expertise. Partnering with universities and educational institutions for internships and workshops can also help bridge this gap.
4. Scalability Constraints:
Challenge: Initial analytics solutions may not be scalable across the organization or adaptable to evolving business needs.
Solution: Choose scalable and flexible analytics platforms from the start. Plan for gradual scaling, starting with pilot projects that can be expanded as the system proves its value and reliability.
5. Cost Overruns:
Challenge: Underestimating the financial investment required for advanced analytics integration can lead to budget overruns.
Solution: Develop a detailed budget that includes not only initial costs but also ongoing maintenance and scaling expenses. Utilize a phased approach to manage expenditures effectively and demonstrate ROI at each stage.
6. Cybersecurity Risks:
Challenge: Increasing data flows and integration raise the potential for cybersecurity threats.
Solution: Strengthen cybersecurity measures by implementing state-of-the-art security protocols and regular audits. Educate staff on cybersecurity best practices and consider cybersecurity insurance to mitigate risks.
By anticipating these challenges and implementing the suggested solutions, supply chain leaders can ensure a smoother transition to advanced analytics-driven operations. Each solution not only addresses specific obstacles but also contributes to building a resilient and forward-thinking supply chain environment.
This blueprint offers a comprehensive roadmap for integrating advanced analytics in supplier selection, enhancing strategic decision-making and operational efficiency. By adopting its methodologies, supply chain leaders can achieve greater cost effectiveness, improve supplier reliability, and enhance overall supply chain resilience, ensuring sustained competitive advantage in a dynamic global market.
Unlock full access to a wealth of resources, including extensive blueprints like this one, by subscribing to SupplyChain360.