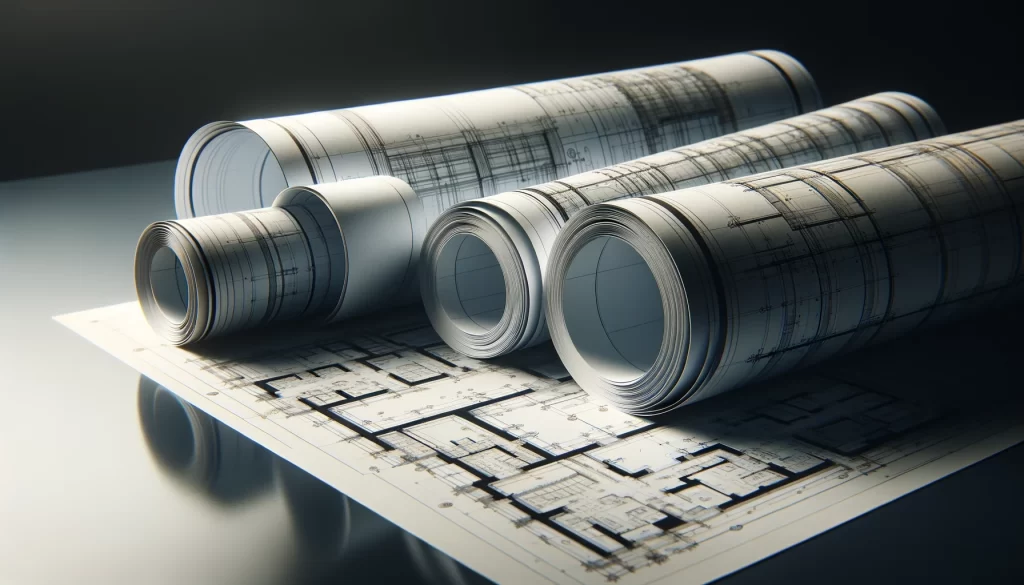
Supply Chain Collaboration and Integration
Blueprints
Supplychain360 blueprints offer an extensive collection of toolkits enabling swift access to best practice to enhance operations or to enable robust decision making.
This blueprint offers a focused path for harnessing strategic alliances to implement AI-driven solutions. It addresses the complex challenge of enhancing resilience and operational efficiency through smart technology. By detailing actionable steps, best practices, and clear metrics, this blueprint provides supply chain leaders with the tools to effectively incorporate AI technologies.
The adoption of these guidelines promises significant improvements in cost-effectiveness, responsiveness, and customer satisfaction, positioning multinational companies at the forefront of industry innovation.
Step-by-Step Implementation Guide: Developing AI-Driven Solutions through Strategic Partnerships
Step 1: Forming Strategic Partnerships
Identify Potential Partners
- Conduct a market analysis to identify technology firms and other multinational companies with advanced AI capabilities.
- Evaluate potential partners based on their technological expertise, market reputation, and alignment with your strategic goals using SWOT analysis.
Establish Partnership Criteria
- Define clear criteria for selecting partners, including technological innovation, scalability of solutions, and cultural fit.
- Utilize the Balanced Scorecard approach to measure potential partners’ performance across multiple dimensions such as financial health, operational efficiency, customer management, and innovation.
Initiate Partnership Discussions
- Arrange meetings with top candidates to explore potential collaboration areas.
- Use the RACI model (Responsible, Accountable, Consulted, Informed) to delineate roles and responsibilities in preliminary discussions.
Step 2: Technology Assessment and Integration
Evaluate AI Technologies
- Review existing AI technologies and tools that can be integrated into your supply chain operations. Focus on solutions for predictive analytics, machine learning for demand forecasting, and automation in logistics.
- Apply the Technology Readiness Level (TRL) framework to assess the maturity of these technologies.
Plan for Integration
- Develop a technology integration roadmap using the Gantt chart to visualize key milestones, including pilot tests and full-scale implementation.
- Consider interoperability with existing systems and ensure that the new AI tools can be integrated without disrupting ongoing operations.
Step 3: Joint Development Initiatives
Structure Co-Development Projects
- Establish joint project teams and define goals using SMART criteria (Specific, Measurable, Achievable, Relevant, Time-bound).
- Implement Agile project management methodologies to allow for flexibility and iterative development in the co-creation of AI solutions.
Manage and Execute Development Projects
- Utilize the Kanban system to manage workflow and ensure continuous delivery of project components.
- Conduct regular sprint reviews and retrospectives to adapt project strategies based on feedback and progress.
Step 4: Rollout and Scaling
Pilot Testing
- Design and implement a pilot test to evaluate the effectiveness of the AI solutions in a controlled environment.
- Use statistical tools like Six Sigma to measure the success of the pilot and identify areas for improvement.
Scaling Strategies
- Develop a scaling strategy based on the Diffusion of Innovations theory to ensure that successful AI solutions are adopted across different departments and locations.
- Establish a change management plan using Kotter’s 8-Step Change Model to guide the implementation and encourage acceptance within the organization.
Best Practices for Implementing AI in Supply Chain Operations
Establish Clear Objectives and Metrics:
- Define specific, measurable objectives for AI implementation, such as improving delivery times, reducing costs, or increasing forecast accuracy.
- Set up key performance indicators (KPIs) to monitor progress and impact, enabling ongoing adjustments to align with strategic goals.
Ensure Data Quality and Accessibility:
- Invest in robust data governance practices to maintain high-quality data. Poor data quality can lead to inaccurate AI outputs, which could compromise decision-making.
- Ensure that data is accessible but secure, with proper data management protocols to facilitate AI applications without compromising data privacy.
Foster Cross-Functional Collaboration:
- Encourage collaboration across different departments—such as IT, operations, and logistics—to integrate AI smoothly into the supply chain.
- Use cross-functional teams to provide diverse insights into how AI can solve practical operational challenges, enhancing buy-in and adoption.
Adopt a Phased Implementation Approach:
- Implement AI technologies in phases, starting with pilot projects that allow for learning and adjustments before full-scale deployment.
- This approach helps in mitigating risks and managing the change more effectively, easing the transition for all stakeholders.
Focus on Continuous Learning and Adaptation:
- AI is not a set-it-and-forget-it solution. Continuously train your AI systems with new data and refine algorithms to adapt to changing market conditions and internal company dynamics.
- Encourage a culture of continuous improvement and learning, where feedback is actively sought and used to improve AI systems and processes.
Leverage Expert Partnerships:
- Collaborate with AI technology providers and consultants who can offer expertise and insights that internal teams may lack.
- These partnerships can provide access to advanced technologies and industry best practices, accelerating AI integration and effectiveness.
By following these best practices, supply chain leaders can maximize the benefits of AI in their operations, driving significant improvements in efficiency, responsiveness, and resilience.
Key Metrics and KPIs for Measuring AI Implementation Success in Supply Chain Operations
Operational Efficiency Improvement:
- Metric: Reduction in order fulfillment time.
- Tracking: Compare historical data on order fulfillment times before and after AI implementation.
- Interpretation: A significant reduction indicates successful AI integration in optimizing logistics and operations.
Cost Reduction:
- Metric: Decrease in logistics and inventory holding costs.
- Tracking: Monitor cost reports and dashboards that integrate financial data from logistics and warehousing.
- Interpretation: Persistent cost declines reflect efficient AI-driven inventory and shipment management.
Accuracy of Demand Forecasting:
- Metric: Improvement in forecast accuracy percentage.
- Tracking: Use statistical software to analyze forecasting errors and compare them against pre-implementation figures.
- Interpretation: Improved accuracy reduces overstock and stockouts, optimizing inventory investment.
Customer Satisfaction:
- Metric: Changes in customer satisfaction and service levels.
- Tracking: Survey customer satisfaction regularly and track service level agreement (SLA) performance metrics.
- Interpretation: Enhanced customer satisfaction and met SLAs suggest that AI is effectively improving customer-facing operations.
ROI from AI Projects:
- Metric: Return on Investment (ROI) for AI projects.
- Tracking: Calculate the net benefits (gains from investment minus cost of investment) divided by the cost of investment.
- Interpretation: A positive ROI indicates that the AI initiatives are adding value beyond their costs.
These KPIs help supply chain directors assess the tangible impacts of AI across various facets of supply chain management, from internal operations to customer interactions, providing a comprehensive view of AI’s contribution to business goals.
Overcoming Implementation Challenges in AI-Driven Supply Chain Solutions
Integration with Legacy Systems:
- Challenge: Integrating advanced AI technologies with outdated legacy systems can be complex and disruptive.
- Solution: Adopt a middleware approach that acts as a bridge between old and new systems, facilitating smoother integration. Plan for gradual phasing out of legacy systems, integrating modular AI enhancements to ensure continuity and minimize disruption.
Data Privacy and Security:
- Challenge: Handling vast amounts of data with AI raises significant privacy and security concerns, especially under stringent regulations like GDPR.
- Solution: Implement robust cybersecurity measures, including encryption and secure data protocols. Regularly update these measures to handle evolving threats. Ensure compliance by consulting with legal experts to adapt AI operations within legal frameworks.
Skill Gaps:
- Challenge: There is often a shortage of skilled professionals who can manage and optimize AI technologies.
- Solution: Invest in training and development programs to upskill existing staff. Alternatively, consider hiring or partnering with AI experts who can bring the necessary expertise to your team. Foster a culture of continuous learning to keep pace with technological advancements.
Scalability:
- Challenge: AI solutions need to be scalable to handle increasing data volumes and expanding business operations without losing performance.
- Solution: Design AI systems with scalability in mind from the outset. Utilize cloud-based solutions to enhance flexibility and scalability. Regular stress testing and system upgrades can also ensure scalability is maintained as the company grows.
Cultural Resistance to Change:
- Challenge: Employees and management may be resistant to adopting AI due to fear of the unknown and potential job displacements.
- Solution: Manage change through transparent communication about the benefits and changes brought by AI. Implement change management practices that include staff in the transition process, offering reassurance and demonstrating how AI will assist rather than replace human roles.
Alignment of AI Strategy with Business Goals:
- Challenge: AI initiatives may not always align with the broader business objectives, leading to inefficiencies and wasted resources.
- Solution: Ensure that AI strategies are developed in close alignment with business goals. Regular reviews and adjustments to AI projects should be conducted in consultation with business leaders to maintain strategic alignment.
By proactively addressing these challenges with the suggested solutions, supply chain leaders can enhance the success rate of AI implementation, ultimately leading to more resilient and efficient supply chain operations.
This blueprint equips supply chain leaders with a strategic approach to integrating AI, enhancing resilience, and driving efficiency. Adopting its guidelines ensures robust partnerships and technological advancements, leading to sustained competitive advantages in the global market.
Unlock full access to a wealth of expertise with a subscription to SupplyChain360. Stay ahead of the curve by exploring more blueprints and exclusive resources tailored for supply chain excellence. Subscribe now and transform your operations with cutting-edge insights and solutions.